Can AlphaFold3 make cancer history?
Maya Kunchur investigates the impact of predictive AI models on the treatment of diseases
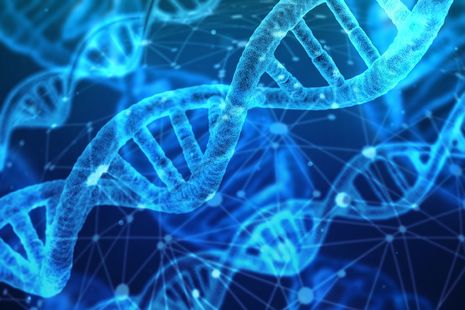
In the 20th century, groundbreaking biological techniques such as DNA sequencing, PCR and new imaging methods permanently changed the face of healthcare. Now, we can see artificial intelligence beginning to do the same. It holds the potential to handle the huge biological datasets generated by technology, and even predict the structure of proteins, potentially unlocking a cure for hundreds of diseases.
Just last week Google’s DeepMinds released AlphaFold3. This updated software uses AI to predict protein structure. These predictions reach an unprecedented degree of accuracy. DNA has a specific sequence of bases, which, like an instruction manual, code for a sequence of amino acids. These amino acids join together in the encoded order and fold in a specific fashion to form a protein. Proteins are fundamental to life and all cellular processes. AlphaFold3 can predict their 3D structure and associated residual groups or nucleotides based on only the input amino acid sequence.
“The cubic millimetre contained around 150 million synapses, so this would be virtually impossible without AI”
Understanding protein structure is crucial to better treat many diseases, including cancer. ‘Cancer’ refers to any disease in which regulation of cell division goes wrong and it therefore becomes uncontrollable, and as such is an incredibly diverse disease. This is partly what makes it so difficult to treat. Understanding protein structures involved in signalling pathways in cell division and how they can go wrong can lead to more specific drug targeting. Additionally, with this tool protein misfolding diseases such as Alzheimer’s and Parkinson’s can have improved biomolecular understanding, making them easier to treat. AlphaFold 3 is just one example of what AI can do for drug development.
Previously, biological data would take a long time to analyse as it would need to be done by hand; using machine learning tools vastly increases efficiency and lowers cost. For example, imaging often produces large amounts of data. AI was recently used to create a 3D map of a piece of a patient’s brain based on image slices. The cubic millimetre contained around 150 million synapses, so this would be virtually impossible without AI. Studying this can help scientists better understand brain diseases that affect millions, such as dementia.
The rapid pace at which AI is developing rightly raises many ethical questions about its potential. In healthcare, one issue is data protection as patient information is very sensitive. Nonetheless, this data already exists regardless of whether it is studied by AI. Another concern is that machine learning is only as good as the dataset it was trained on, which can easily lead to biases. For example, if an AI tool were used to compare SNPs (short sections of DNA which are often used to track disease inheritance) across populations, it would need to differentiate SNPs which are more likely to be associated with a particular disease depending on the ethnic ancestry of the patient. Moreover, the expected healthy range for a particular characteristic may depend on attributes like the patient’s sex, so AI must be trained on a dataset with even representation. However, obtaining a completely representative data sample is very difficult.
“AlphaFold3 trials showed accuracy up to 76%. While this is groundbreaking, an expert would still be required to manually filter AI results based on their knowledge, or experiments”
It is time-consuming to ensure there is a large enough manually-labelled dataset for AI to draw from. Some projects use open-source platforms simply for sheer numbers of manual ‘trainers’ for their machine learning tools: Zooniverse is an online platform where anyone can contribute to scientific projects, including by helping to train AI.
These drawbacks are incredibly important to consider when AI is being applied to medical fields, because inaccuracies such as an incorrect diagnosis or a missed disease could be fatal. This is why it looks like AI will simply become a small part of medical professionals’ toolkits for the near future, still requiring a manual operator. AlphaFold3 trials showed accuracy up to 76%. While this is groundbreaking, an expert would still be required to manually filter AI results based on their knowledge, or experiments.
But the inaccuracy can be exploited. If AI were, for example, too sensitive at picking up a particular disease, it could still be beneficial as an early-warning system which indicates to medical professionals that they may need to carry out more tests – such as with recent electrocardiogram (ECG) trials carried out by the National Defence Medical Centre in Taiwan. AI studied ECGs and flagged them to medical professionals. Compared to a control group, this resulted in a lower mortality rate in high-risk patients.
The potential for AI in healthcare is limitless. Over our lifetimes, diagnostics and the level to which diseases can be studied will be transformed by machine learning. However, there is a considerable way to go before medical professionals no longer make the final call.
News / Caius clock hand returned nearly 100 years after student prank
31 March 2025News / Hundreds of jobs to be cut at Cambridge University Hospitals
1 April 2025Comment / More Cambridge students should study abroad
1 April 2025Features / Cloudbusting: happy 10th birthday to the building you’ve never heard of
30 March 2025Fashion / The Cambridge puffer: a debate
27 March 2025