How medical research is failing women – and how to fix it
Jade Scardham examines whether AI has the potential to resolve the gender data gap
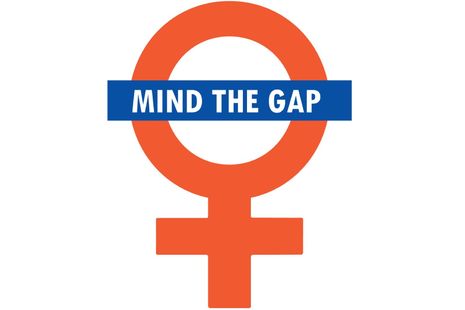
The gender data gap refers to the systemic lack of data relevant to the experiences of women and girls. This is particularly pronounced in healthcare, as women are frequently underrepresented in medical research, and data isn’t often collected separately for men and women. This data gap leads to misdiagnoses, ineffective treatments, and worse health outcomes for women. Women’s symptoms are often ignored or misunderstood, harmful side effects are increased because drug dosages are based on male physiology, and diseases like heart conditions and autoimmune disorders are often missed or under-treated in women. The use of artificial intelligence (AI) could help close the gender data gap by finding overlooked patterns in women’s health, but it could also risk widening inequalities if it is trained on biased data.
There are two main factors contributing to the gender data gap. On one hand, diseases that mostly affect women are under-researched due to exclusion from clinical trials and lack of funding. At the same time, medical evidence is often interpreted from a male-centric perspective, leading to incorrect assumptions about how diseases manifest and should be treated in women. These biases skew public health data and medical progress, resulting in poor healthcare policies, delayed diagnoses, and a lack of medical innovations for women. There has also historically been a failure to consider aspects of women’s health outside of reproductive issues.
“Medical evidence is often interpreted from a male-centric perspective”
Women are often misdiagnosed or the severity of their symptoms overlooked due to biases and gaps in medical training. For example, women are 50% more likely than men to be misdiagnosed during a heart attack because their symptoms often differ from men’s and are often attributed to gastrointestinal or anxiety-related symptoms. Similarly, ADHD in women and girls is frequently overlooked because symptoms can present differently than in men and boys. Gender bias in healthcare contributes to women’s chronic pain being attributed to psychological causes rather than physical ones, resulting in inadequate pain management. Endometriosis takes an average of seven years to diagnose due to medical dismissal and lack of focus on women’s pain.
Many countries don’t collect or report health data separately for men and women (referred to as sex-disaggregation), making it hard to track disparities in disease prevalence, treatment effectiveness and healthcare access. Current health metrics underestimate the impact of menopause, endometriosis, and other chronic conditions, failing to reflect their effects on women’s quality of life and economic productivity.
Women have long been excluded from clinical trials, leaving medical research focused on men. This leads to a lack of specific analysis and treatments that don’t fully address women’s health needs. The indiscriminate application of research based on men to women runs the risk of ignoring differences that affect disease progression, symptoms and treatment.
To improve this situation, healthcare institutions should focus on more inclusive research and medical training, and fair diagnostic protocols to ensure women get accurate and timely care. Policymakers must incentivise women’s inclusion in clinical trials and more nuanced data collection, and fund research on diseases that disproportionately affect women.
AI tools have the potential to transform healthcare by analysing large amounts of medical data and uncovering patterns that traditional research has missed. Researchers have already developed machine learning algorithms that can be used to analyse mammograms with high accuracy. Similar tools could improve diagnosis by identifying subtle differences in symptoms between women and men, reducing the risk of misdiagnosis. AI screening models can also analyse patient history, genetic markers, and clinical data to create personalised treatment plans, raising the possibility of therapies that are tailored to an individual’s physiological and hormonal profile. This would improve early diagnosis, treatment effectiveness, and long-term health outcomes for women.
“If trained on biased data, AI can worsen healthcare gaps by reinforcing existing exclusionary patterns”
However, if trained on biased data, AI can worsen healthcare gaps by reinforcing existing exclusionary patterns. For example, a study by UCL researchers found that AI screening tools for liver disease were nearly twice as likely to miss diagnoses in women compared to men. This discrepancy happened because the AI models relied on biochemical markers, such as lower albumin levels, which are more effective indicators of liver disease in men than women. AI models need to account for differences in disease presentation and treatment response to ensure equitable healthcare outcomes, rather than reinforcing male-focused medical norms that neglect women’s health needs. Developers must tackle bias by using diverse training data, including gender-specific health factors and implementing transparency measures.
Inclusive research should become the standard, ensuring that women are properly represented in clinical trials, and that studies consider variations in disease symptoms, progression, and treatment response. More funding for inclusive health research is essential to close longstanding gaps, especially in conditions where women are underrepresented. Medical research and public health reporting should analyse data separately for men and women where appropriate to uncover disparities, improve treatment effectiveness, and drive more equitable healthcare outcomes.
Closing the gender data gap isn’t just about justice – it improves healthcare by leading to better diagnostics, treatments, and medical breakthroughs for everyone.
Want to share your thoughts on this article? Send us a letter to letters@varsity.co.uk or by using this form.
News / Cambridge student numbers fall amid nationwide decline
14 April 2025News / Greenwich House occupiers miss deadline to respond to University legal action
15 April 2025Comment / The Cambridge workload prioritises quantity over quality
16 April 2025Sport / Cambridge celebrate clean sweep at Boat Race 2025
14 April 2025Features / The TikTok college: using social media in access and outreach
15 April 2025