AI in scientific research: friend or foe?
Teymour Taj weighs up the opportunities and challenges that AI poses for the scientific community
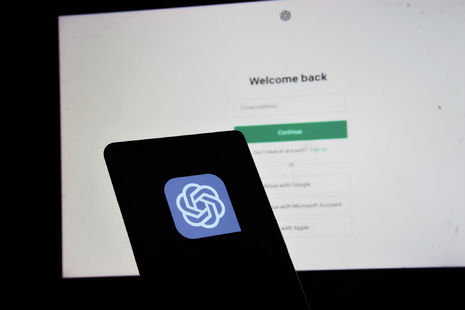
Artificial intelligence has completed the transition from sci-fi film buzzword into a feature of everyday life. An open ChatGPT tab can now be found on every stressed-out university student’s browser. The proliferation of AI technology has inevitably also made its way to scientific research, presenting great opportunities for the advancement of our understanding of the world, but also a series of complex ethical challenges.
According to analysis of the Scopus database, the proportion of articles published in research papers citing AI has risen from around 2% in 2013 to 8% just ten years later. Artificially intelligent algorithms are being used in a wide range of scientific contexts; for example, AI is being heavily implemented in molecular biology to solve the protein folding problem, enabling us to understand the structure of the 300 million known proteins simply from a short section of genetic code. If a solution is found, it would represent one of the greatest modern-day advances in scientific knowledge, leading to limitless advances in curing and treating disease, producing new biomaterials and preventing future pandemics.
Programs such as AlphaFold, created by Google’s parent company, are using machine learning techniques to make more accurate predictions of the shapes of different proteins. AlphaFold is trained on a public database of around 100,000 proteins, comparing the amino acid sequences determined by the genetic code to the known structures of the proteins. A 2021 paper published in Nature suggested that the predictions were highly accurate down to the scale of a single carbon atom: the paper has since become one of the most cited of all time. The AlphaFold model has already been used to develop drugs, find enzymes to break down polluting plastics, and even produce vaccines against previously elusive diseases such as malaria.
“Clearly, AI has the potential to transform scientific research and find solutions to age-old, highly complex computational problems”
In addition, in 2020 Exscientia produced the first ever AI-discovered drug to enter a clinical trial, aimed at treating OCD symptoms. Their program searched through extremely large chemical libraries at record pace in order to identify molecules that could potentially target the right receptors to treat the disorder. Typically, drug development by clinical scientists is a process that takes many years to complete. There is usually an interval of about five years just from the initial screening of molecules to the beginning of clinical trials. However, for Exscientia’s drug, this stage was completed in less than a year.
Clearly, AI has the potential to transform scientific research and find solutions to age-old, highly complex computational problems much faster than a human or regular computer ever could. However, I believe that we must be cautious about how widely AI is used within research, and how much we trust content generated by such programs.
Current AI models are trained on sets of data which already exist, never far from human influence. Although large sets of numbers may seem to be objective, there are always biases present in the collection, processing and reporting of data. For example, clinical trial data has long been known to overrepresent white patients. We know that certain conditions that affect our reaction to drugs, such as diabetes and sickle cell disease, vary in prevalence between population groups of different descent. Hence, an algorithm trained on this data will absorb this overrepresentation and may suggest drugs which have different effects on people from ethnic minority backgrounds, a shocking example of algorithmic bias. This raises difficult questions about the ethics of using AI in research. Should a computer algorithm be subject to the Equality Act and held to the same legal standards as humans? And if found in breach, who is responsible?
“Algorithms have no understanding of what the data represents in the same way that a human researcher does”
This issue has come to the fore recently, with the Metropolitan police announcing that they will use facial recognition technology on London’s streets to identify suspected criminals. However, the algorithm used was reportedly trained using predominantly white faces, and a study from the University of Essex found it was only accurate in 19% of cases, compared to the Met’s claimed figure of 70%.
One suggested solution for these issues has been a rigorous vetting process for machine learning models, involving direct testing of the models against known data to detect bias against certain groups. The developers could also be made to produce a bias impact statement, outlining which groups might be affected, and how bias against them would be detected. This would be difficult to enforce, though: proving that a model is biased against a particular group is challenging when there are a near-infinite number of confounding factors to account for.
In addition, machine learning algorithms rely on finding patterns in the datasets they have been trained on, and extrapolating those patterns to new data. Algorithms have no understanding of what the data represents in the same way that a human researcher does. Hence these algorithms cannot distinguish between patterns due to noise in the data, and actual trends in the data, potentially generating incorrect predictions.
Overall, the increasing use of AI can be positive for scientific research. It has the potential to revive research on unanswered questions where no breakthroughs have been made in years, spurring a modern-day scientific revolution. Nevertheless, research produced by artificially intelligent models should be treated with a pinch of salt. It is clear that they can reinforce existing human biases under a veneer of objectivity: strict scrutiny of the information used to train machine learning is required, and the propagation of AI in research should be controlled until adequate regulation can be put in place.
Comment / How a culture of knowing shapes the Cambridge application process
1 March 2025News / Murder investigation launched following Chesterton Road stabbing
27 February 2025Theatre / L’incoronazione di Poppea: lessons in power and patience
1 March 2025News / Robinson swaps May Ball for a ‘mega bop’
26 February 2025News / Pro-Palestine protesters hold rally during graduation ceremonies
2 March 2025